As data centres push to prepare their facilities for AI use cases, memory and storage remain the misunderstood and overlooked aspects of hardware deployments. How does AI memory and storage work, why is it so important to get it right and how should data centre leaders be approaching it? Jeff Potter, Senior Manager Marketing Operations at ECS (Equus Compute Solutions), shares his insights.
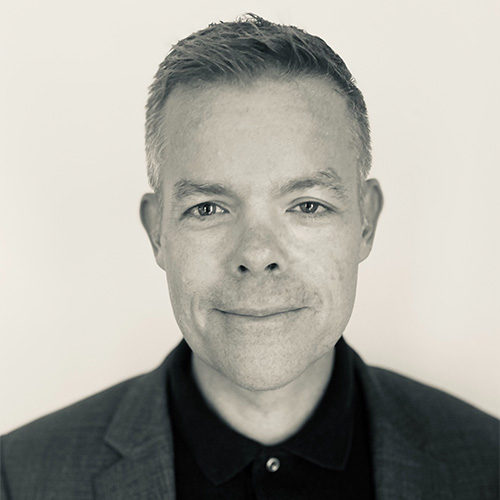
Generative AI has captured the imagination and interest of people worldwide. Unlike traditional AI systems, Generative AI creates content – text, images, videos – by learning from vast amounts of data. This leap from data analytics to content creation has brought AI from the realms of data scientists to everyday conversations. Today, even those not deeply versed in technology know about AI, largely thanks to platforms like ChatGPT.
The recent boom in interest in AI highlights Generative AI’s practical utility, showcasing how it can democratise programming and expand creative possibilities for non-experts.
In response, data centres are scrambling to get a handle on this approaching horizon of AI deployments, pushing for new ways of designing and deploying racks that will serve this new purpose. Amid this process, it can be tempting to focus on algorithms and computing power at the core of AI efficiency.
An often-overlooked aspect of AI deployments, however, is memory and storage. Why does AI rely so heavily on memory and storage, how does it integrate into AI deployments in a new way, and how should data centres be thinking about it to maximise AI efficiency in their IT infrastructure?
The data backbone of AI
First, to understand why storage and memory are so vital, let’s take a step back to evaluate exactly how data flows through AI in the first place. The AI process involves three primary stages.
To begin, there is capturing, the collection of information from a wide range of sources including devices, sensors and user inputs. Next, the data must be organised. This process involves structuring, sanitising and labelling the collected data in a way that ensures data quality for correct and unbiased outcomes.
The final step is utilising the data, which is the stage that gets the most attention, and rightly so. Data utilisation through AI models comes in two flavours: training and inference. Training involves using large datasets to teach AI models to recognise patterns and generate new data. Inference, on the other hand, applies the trained model to new data, generating responses or predictions based on what the model has learned.
Understanding the difference between training and inference is key to grasping AI’s operational intricacies. Training is complex and resource-intensive, requiring substantial memory, storage and computational power to process vast amounts of data. It can take days or weeks to complete. Inference, on the other hand, requires less computational burden to provide outputs. It happens in real-time so it depends far less on powerful hardware but far more on speedy hardware. Balancing these two elements is crucial for designing and deploying AI systems efficiently, and underscores AI’s reliance on advanced memory and storage solutions.
Optimising AI hardware for data management
The core of AI data management is ensuring equilibrium between data mass and velocity, maintaining an efficient system between training and inference. In this way, the most challenging aspect of AI infrastructure design is achieving seamless data flow, a task that cannot be left to chance. In this context, the significance of storage and memory in AI applications is critical, yet often understated. CPUs and GPUs handle the bulk of the computational load, so they are often the recipient of much consideration. However, the efficiency of the processor depends on how quickly and consistently it can access data. In this way, the accuracy of AI inferencing and the speed of AI training is only as good as the servers supporting it.
It may seem like stating the obvious to say that choosing the best servers for the job is important for a new deployment, but for AI getting the servers right is even more important than usual. This is because AI use cases utilise local storage as caches for delivering training data into High Bandwidth Memory (HBM) on a given processor. Consequently, the performance of the AI application hinges on a server with advanced memory solutions designed for high throughput.
On top of that, these local storage caches are highly responsible for accelerating data access to GPUs, so servers must also facilitate fast and continuous operational flow, in addition to high-volume operational flow. If networked data lakes aren’t powered by strong, high-capacity storage, data will bottleneck because the AI servers are not fed with enough data to keep everything mostly swiftly and smoothly. In sum, we can see how equipping cabinets for AI applications with cutting-edge memory and storage hardware can greatly shorten AI model training times, reduce computing expenses and improve the precision of AI inference.
The memory and storage pyramid
So, if AI relies so heavily on memory and storage infrastructure, how exactly can data centres set up their systems to maximise efficient data movement from storage to processing units? It’s helpful to think of the deployment as a pyramid, with near-memory components at the top and network data lakes at the base.
At the top of the pyramid is high-bandwidth memory that can handle HBM and graphics double data rate GDDR SRAM, residing closest to the computing units to ensure rapid data access. The second layer of the pyramid is a bit larger than the top and is reserved for main memory like DDR4 and DDR5 SRAM.
Just below that in the hierarchy is expansion memory, a new memory class allowing expansion beyond traditional memory limits that utilises compute express link (CXL) specifications.
Nearing the bottom of the pyramid, the fourth (and second-largest) layer is local SSDs. Their high data rates play a crucial role in local caching and data access. At the base of the pyramid are network data lakes, necessary for training AI models in a way that balances performance and cost-effectiveness.
The way the pyramid works is that near-memory solutions sitting at the apex provide fast data access while the main memory feeds data to the GPUs. Meanwhile, expansion memory ensures scalability (starting at terabyte levels). These technologies work on top of local SSDs and network data lakes forming the base to store vast amounts of data. All in all, this pyramid setup is the key to effective AI deployments in a way that maximises training and inference processes concurrently.
The future of AI and storage
The often-underestimated role of memory and storage is critical in optimising AI deployments. But, by understanding the intricacies of data flow and leveraging advanced memory and storage solutions, data centres can significantly enhance the efficiency and effectiveness of AI applications. Keeping an eye on AI training and inference simultaneously, data centre leaders can employ a pyramid strategy to not only reduce lag time and computing costs but also improve the accuracy of AI processing itself. As AI continues to evolve, this well-balanced and designed infrastructure will be essential for amplifying AI’s potential in data centres, driving industry innovation now and in the future.